Powering the AI Revolution: Meeting the Demands of Next-Gen Workloads
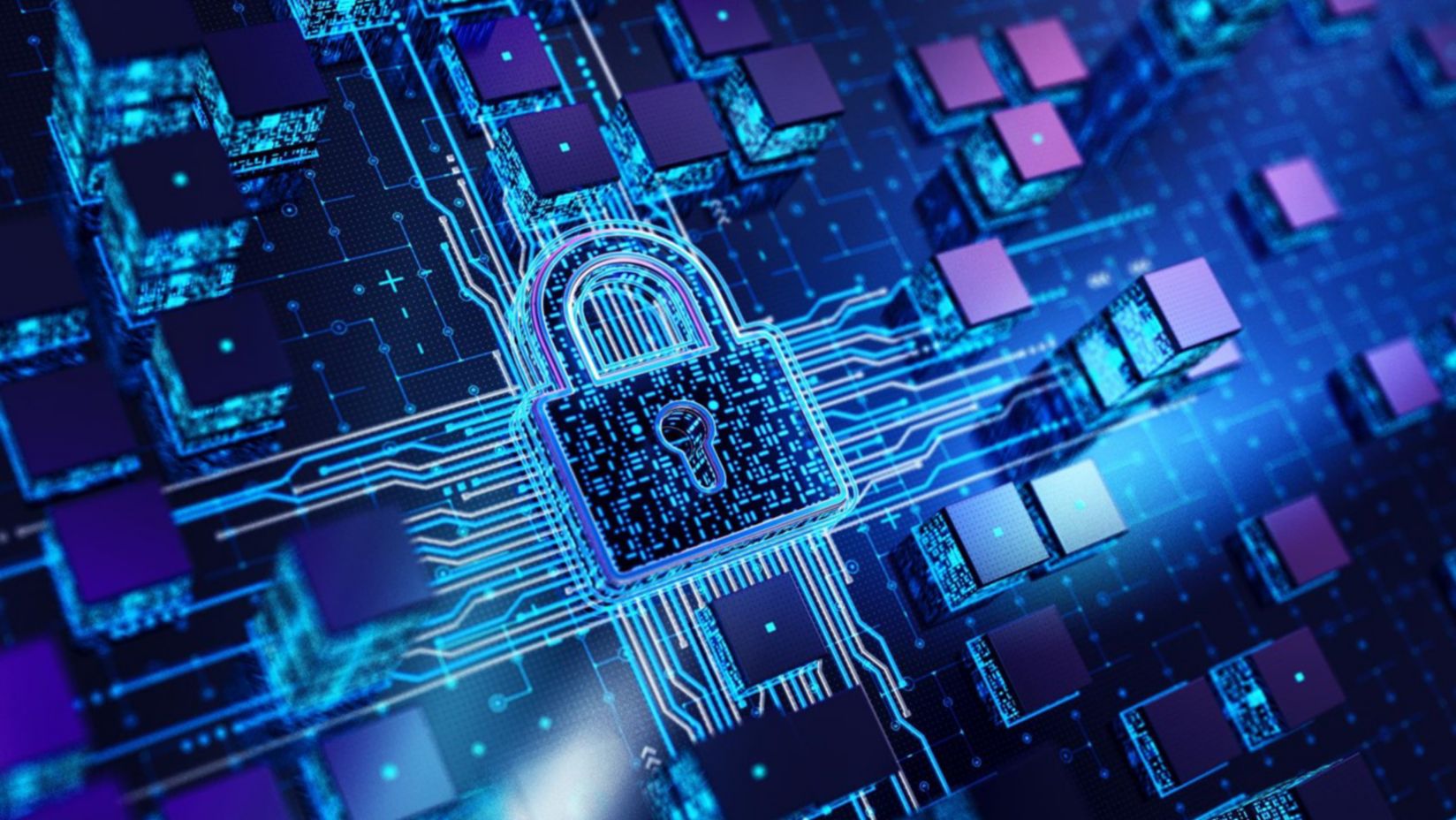
The rapid rise of generative AI is fuelling an unprecedented expansion of data centre capacity, with intensive workloads often demanding significantly higher power densities than traditional compute environments. Meanwhile, Gartner highlights four major trends shaping the future of cloud, data centre, and edge infrastructure, all of which point to the need for more robust, scalable, and efficient operations.
For CIOs, CTOs, and infrastructure architects, the stakes are clear: without modernised infrastructure, AI deployments will face performance bottlenecks, rising costs, and security risks. But what constitute a modernised infrastructure? Let’s look at some features and key considerations.
1. Power and cooling: why AI needs more juice
AI workloads aren’t just compute-heavy—they’re power-hungry. Unlike traditional IT racks that consume 8.4 kW, AI racks now routinely exceed 30 kW, with some reaching 80 kW per rack. As organisations shift from CPU-driven environments to AI accelerators such as GPUs and TPUs, power consumption spikes alongside the heat these processors generate.
Why this matters:
AI’s increasing power density is straining legacy data centres that rely on air cooling, creating thermal inefficiencies, higher costs, and downtime risks.
Key considerations
Advanced cooling techniques: The reliance on traditional air cooling has limited viability for AI workloads. The surge in generative AI usage will likely further intensify the focus on sustainable data centre designs, including liquid cooling and energy recovery systems.
Future-ready rack densities: AI deployments can easily surpass 30 kW per rack, making data centres with higher-capacity cooling and power delivery an absolute necessity.
2. Security and compliance: protecting your competitive edge
AI models represent competitive advantage, intellectual property, and proprietary data—making them prime targets for cyberthreats. Without robust security measures, businesses risk IP theft, regulatory penalties, and operational disruptions.
Why this matters:
- AI training datasets often contain sensitive customer, financial, or healthcare data, which require strict data sovereignty compliance.
- AI models and inference engines can be vulnerable to adversarial attacks, which leads to compromised decision making.
Mitigation tactics
Physical and network segmentation: Implement biometric access, 24/7 surveillance, and strict partitioning of AI training environments from broader corporate IT systems.
Regulatory preparedness: As AI becomes more pervasive in regulated industries, data centres offering ISO 27001, SOC 2, and other relevant certifications help simplify compliance.
Targeted cloud interconnects: Private, direct links to public cloud environments allow for elasticity without sacrificing data governance and security controls.
3. Scalability and network connectivity: fuelling real-time intelligence
AI thrives on massive datasets, but only if data can move fast enough to meet real-time demands. AI applications, from autonomous vehicles to financial risk modelling, require ultra-low-latency processing and massive bandwidth scalability.
Why this matters:
- AI model training requires moving petabytes of data across compute clusters—any delay slows innovation.
- Inference at the edge needs real-time processing, requiring AI stacks to be deployed closer to data sources.
Actions to consider
Proximity to cloud hubs: Locating workloads in or near major cloud regions cuts round-trip times.
High-bandwidth options: For training clusters involving petabytes of data, 100G or 400G interconnects may be necessary to prevent throughput bottlenecks.
Edge readiness: AI at the edge requires smaller, distributed data centres capable of rapid scaling and local data processing. This approach reduces latency for use cases like industrial IoT, vehicle autonomy, and immersive digital experiences.
4. Uptime and expertise: keeping AI always-on
AI infrastructure must be resilient, redundant, and supported 24/7—any downtime can disrupt real-time analytics, autonomous operations, and business-critical AI applications.
Why this matters:
- AI workloads are always-on, requiring high availability and real-time failover.
- Downtime costs enterprises millions in lost productivity, service outages, and reputational damage.
Ensuring resilience
High uptime SLAs: Redundant power feeds, N+1 cooling, and multiple network carriers help data centres achieve 99.99% availability or higher.
Geographic redundancy: Replicating data or setting up automated failover in another region can shield operations from localized outages, whether natural disasters or network faults.
Dedicated support: Immediate, around-the-clock assistance is crucial for resolving issues in real time—critical when AI workloads can’t afford to be offline.
How colocation fits in
As AI deployments scale, colocation provides a flexible, specialised environment that can handle high-power, high-density racks without forcing enterprises to build massive, capital-intensive private data centres. This model also offers:
Shared economics and expertise: Colocation leverages a facility designed specifically for high-performance workloads. Operational costs for advanced cooling, connectivity, and security are spread among tenants.
Customisable scalability: Companies can scale up (or down) as AI needs evolve, with racks being reconfigured without a complete infrastructure overhaul.
How to get started
- Audit your AI footprint: Identify which workloads will need high-performance accelerators and gauge how quickly they might grow.
- Align on sustainability: Consider whether liquid cooling, green energy sourcing, and energy-efficiency commitments are goals in your business model.
- Plan for resilience: Map out fallback scenarios for hardware failures, power outages, and region-wide disruptions to maintain continuous AI operations.
Data centre capacity must scale in lockstep with the inexorable growth of AI-driven services. This requires forward-looking solutions that address both performance and sustainability. By leveraging modern colocation, enterprises can ensure they’re ready to harness AI’s transformative power without being constrained by legacy infrastructures.
Future-proof your AI infrastructure. Don’t let power, cooling, or latency bottlenecks stall your AI ambitions.
Colocation services provider checklist – Accelerate cloud modernisation and prepare for the future with the right partner.
Schedule an assessment – Get an infrastructure strategy tailored for AI-scale workloads.
The capabilities and specifications mentioned in this article do not apply to all owned, managed, and resell data centres under Telstra International’s colocation services. Please enquire for detailed facility specifications and availability.